Types of Intelligent Agents Explained Simply
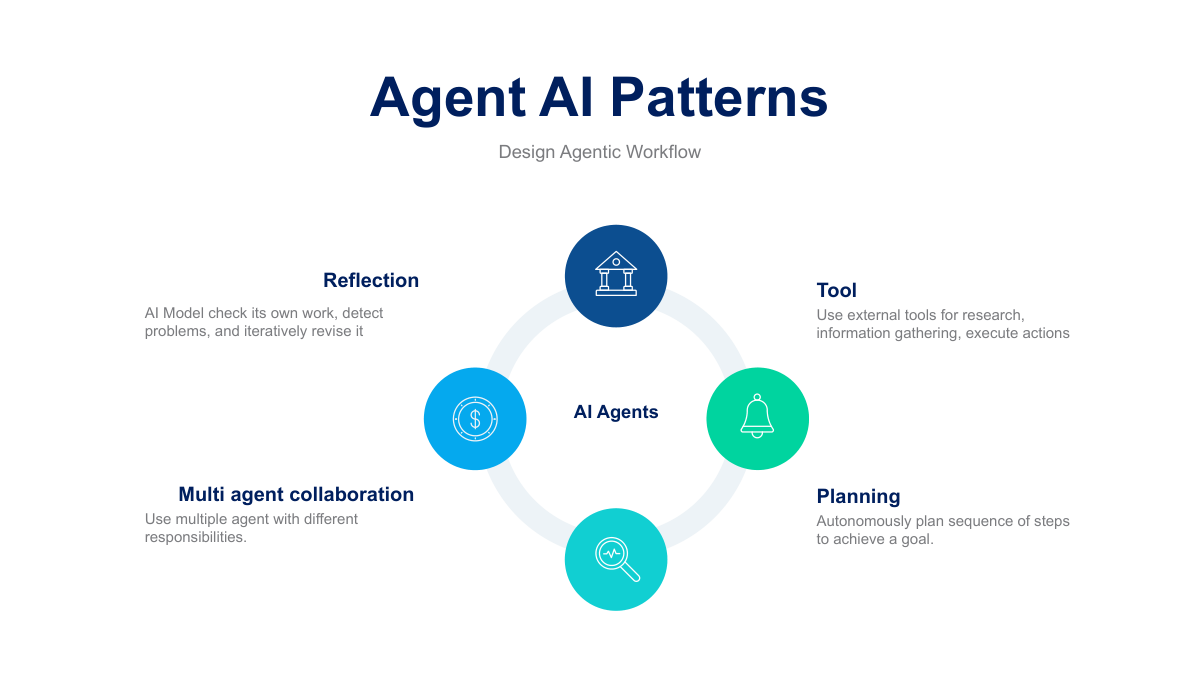
|
||||||||||||||||||||||
|
||||||||||||||||||||||
Dataknobs has developed a wide range of products and solutions powered by Generative AI (GenAI), Agent AI, and traditional AI to address diverse industry needs. These solutions span finance, healthcare, real estate, e-commerce, and more. Click on to see in-depth look at these use cases - Stocks Earning Call Analysis, Ecommerce Analysis with GenAI, Financial Planner AI Assistant, Kreatebots, Kreate Websites, Kreate CMS, Travel Agent Website, Real Estate Agent etc.
DataKnobs has built an AI Agent for structured data analysis that extracts meaningful insights from diverse datasets such as e-commerce metrics, sales/revenue reports, and sports scorecards. The agent ingests structured data from sources like CSV files, SQL databases, and APIs, automatically detecting schemas and relationships while standardizing formats. Using statistical analysis, anomaly detection, and AI-driven forecasting, it identifies trends, correlations, and outliers, providing insights such as sales fluctuations, revenue leaks, and performance metrics.
Here are slides and AI Agent Tutorial. Agentic AI refers to AI systems that can autonomously perceive, reason, and take actions to achieve specific goals without constant human intervention. These AI agents use techniques like reinforcement learning, planning, and memory to adapt and make decisions in dynamic environments. They are commonly used in automation, robotics, virtual assistants, and decision-making systems.
Building data products using Generative AI (GenAI) and Agentic AI enhances automation, intelligence, and adaptability in data-driven applications. GenAI can generate structured and unstructured data, automate content creation, enrich datasets, and synthesize insights from large volumes of information. This helps in scenarios such as automated report generation, anomaly detection, and predictive modeling.
At its core, KreateHub is designed to enable creation of new data and the generation of insights from existing datasets. It acts as a bridge between raw data and meaningful outcomes, providing the tools necessary for organizations to experiment, analyze, and optimize their data processes.
CIOs and CTOs can apply GenAI in IT Systems. The guide here describe scenarios and solutions for IT system, tech stack, GenAI cost and how to allocate budget. Once CIO and CTO can apply this to IT system, it can be extended for business use cases across company.
Here are several value propositions for Retrieval-Augmented Generation (RAG) across different contexts: Unstructred Data, Structred Data, Guardrails.
See Drivetrain appproach for building data product, AI product. It has 4 steps and levers are key to success. Knobs are abstract mechanism on input that you can control.