Dimensions of Vector DB | Slide
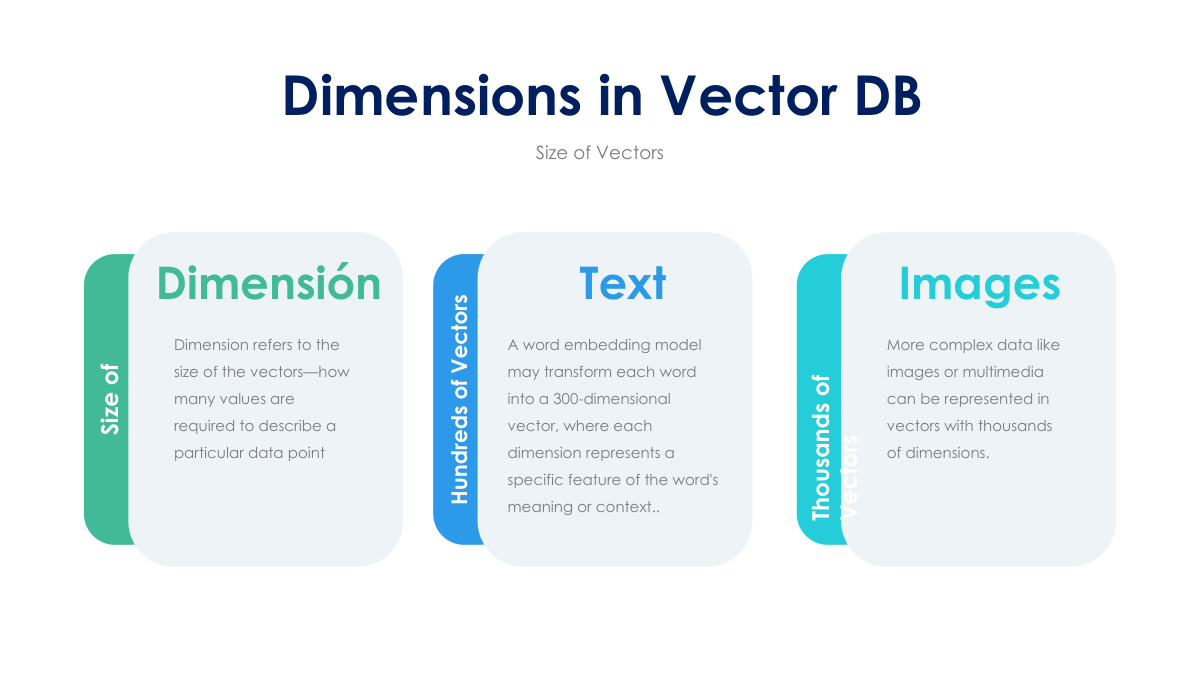
|
||||||||||
Detail Article |
||||||||||
A Deep Dive into Vector Databases and Their Role in Modern AI ApplicationsIn the rapidly evolving world of artificial intelligence, data-driven technologies like Vector Databases (Vector DBs) are transforming how businesses manage and leverage information. Vector DBs are becoming a key enabler for a wide range of AI-powered applications, from improved search algorithms to sophisticated retrieval-augmented generation (RAG) scenarios used in AI assistants. This article will delve into what vector databases are, how data scientists utilize them, and how business executives and managers can understand and leverage this technology for their own organizations. What Are Vector Databases?At their core, vector databases are specialized databases designed to store and query high-dimensional vectors—numerical representations of data points. These vectors are typically generated through machine learning models like word embeddings, image encoders, or graph-based neural networks, which convert complex, unstructured data (such as text, images, and videos) into numerical forms that machines can easily process. A typical database stores data in rows and columns. In contrast, vector databases are optimized for searching through large collections of high-dimensional vectors, often in hundreds or even thousands of dimensions. This is especially useful for scenarios where exact matches aren't needed, but rather "similar" items are sought—think of image recognition systems, recommendation engines, and semantic search applications. Dimensions in Vector DBsWhen we talk about dimensions in a vector database, we're referring to the size of the vectors—how many values are required to describe a particular data point. For instance, a word embedding model may transform each word into a 300-dimensional vector, where each dimension represents a specific feature of the word's meaning or context. Larger, more complex data like images or multimedia can be represented in vectors with thousands of dimensions. The effectiveness of vector databases depends on the careful selection of the number of dimensions, balancing computational complexity with the precision of search results. For data scientists, understanding how to manage and optimize these dimensions is crucial for effective model training and fast querying. How Data Scientists Use Vector DBs for Search and AI Applications
How Business Executives and Managers Should Understand Vector DBsFor business executives and managers, the technical complexity of vector databases might seem daunting. However, it's important to focus on the transformative value these databases bring to a variety of business applications:
Key Considerations for Business LeadersWhile vector databases offer immense potential, executives must consider several factors before implementing them:
ConclusionVector databases represent a revolutionary shift in how businesses can store, search, and utilize high-dimensional data. For data scientists, these databases are invaluable tools for powering AI applications like semantic search, recommendation systems, and RAG scenarios. For business executives and managers, the key to success lies in understanding how these technologies can enhance customer experiences, streamline operations, and unlock new business opportunities. By grasping the strategic value of vector databases and fostering collaboration between technical and non-technical teams, organizations can stay ahead in today’s AI-driven economy. |
||||||||||